Retrieval-augmented generation
Optimize your data for generative AI
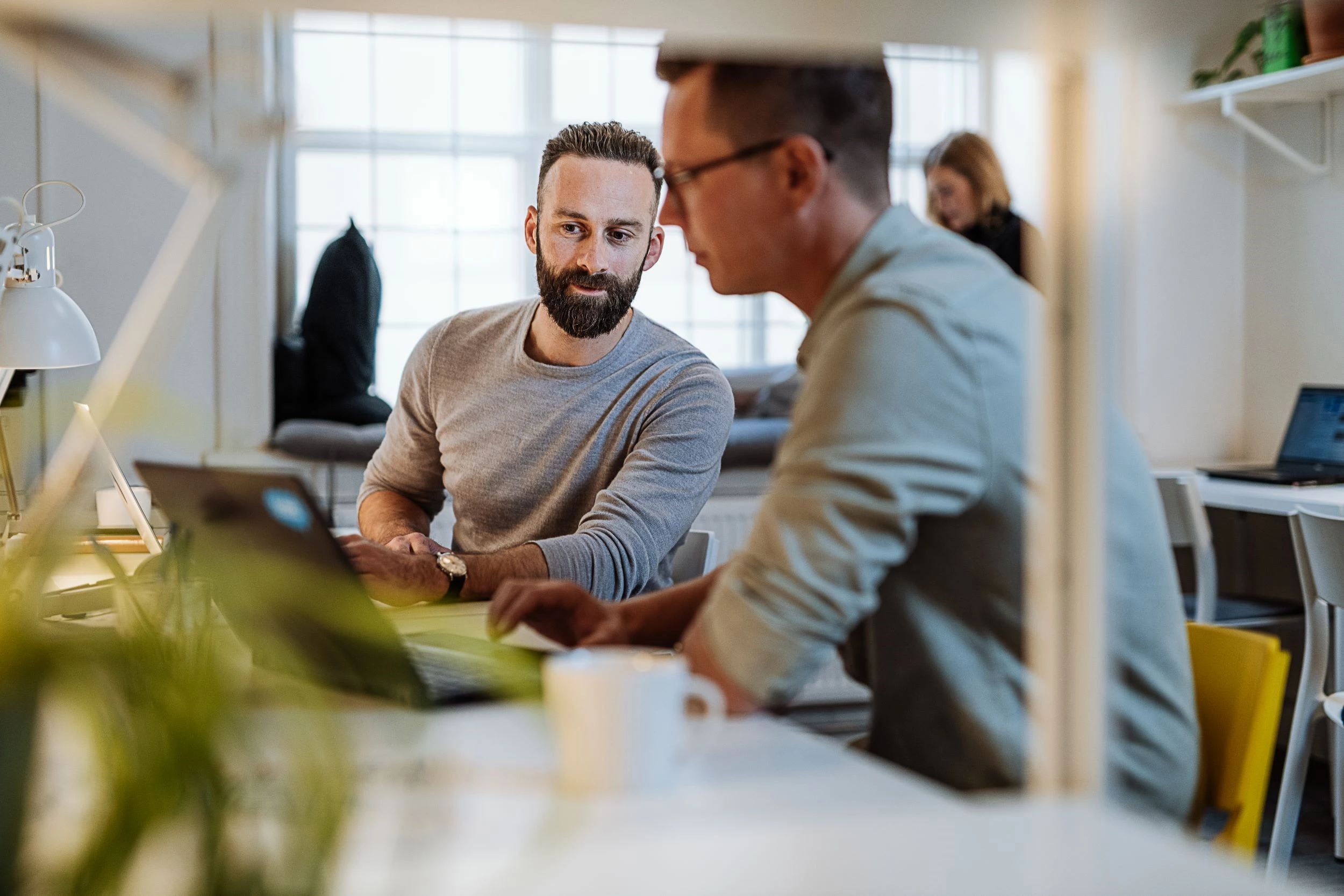
What is retrieval-augmented generation (RAG)?
Retrieval-augmented generation (RAG) is a cutting-edge Al methodology that optimizes the accuracy and quality of LLMs by connecting them to external knowledge sources.
Large language models (LLMs) have revolutionized content generation, but their responses aren't always consistent. They're only as dynamic and relevant as the data used to train them.
With impeccable data delivered through purpose-built AI powering your RAG technology, your LLM will dynamically pull information from a vast external text database, based on each query. This gives the model access to the most current, verifiable facts. It also allows for more nuanced and context-rich answers, which is particularly valuable in sectors that require in-depth topic knowledge.
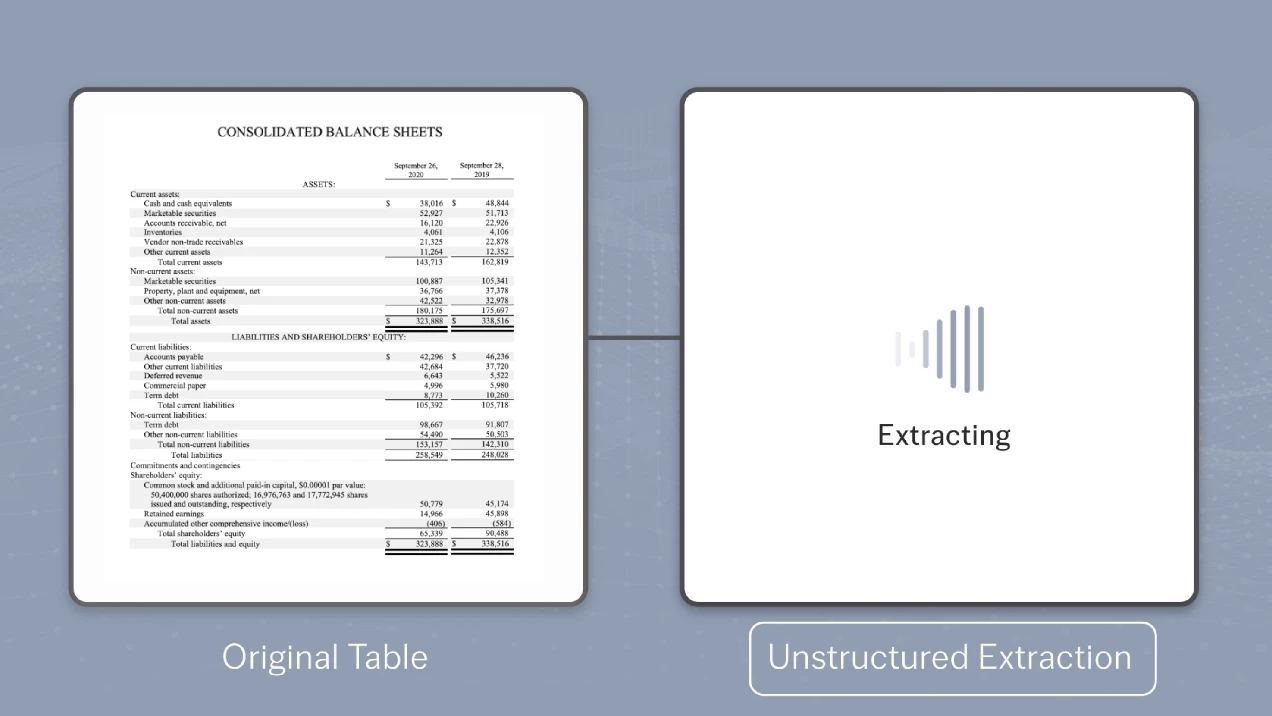
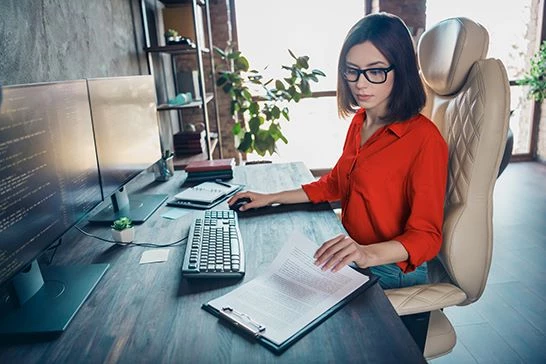
Transform hidden data into valuable insights
Today, 90% of business data is stored in formats that challenge traditional “extract, transform, load” (ETL) processing. These formats include PDF, TIFF, PNG, PPTX, or DOCX. This level of data inaccessibility hinders complete business transformation.
We leverage purpose-built AI to help you extract meaningful insights from any type of document. Vantage, our intelligent document processing platform, uses advanced AI techniques to extract, classify, and deliver data from documents. By integrating Vantage, your document data enables enriched and more relevant insights, based on a broader knowledge base for your LLM.
The power of retrieval-augmented generation
Use purpose-built AI to generate high-quality data that fuel your RAG system for successful generative Al implementations.
Efficient training
Reduced bias
Enhanced contextual understanding
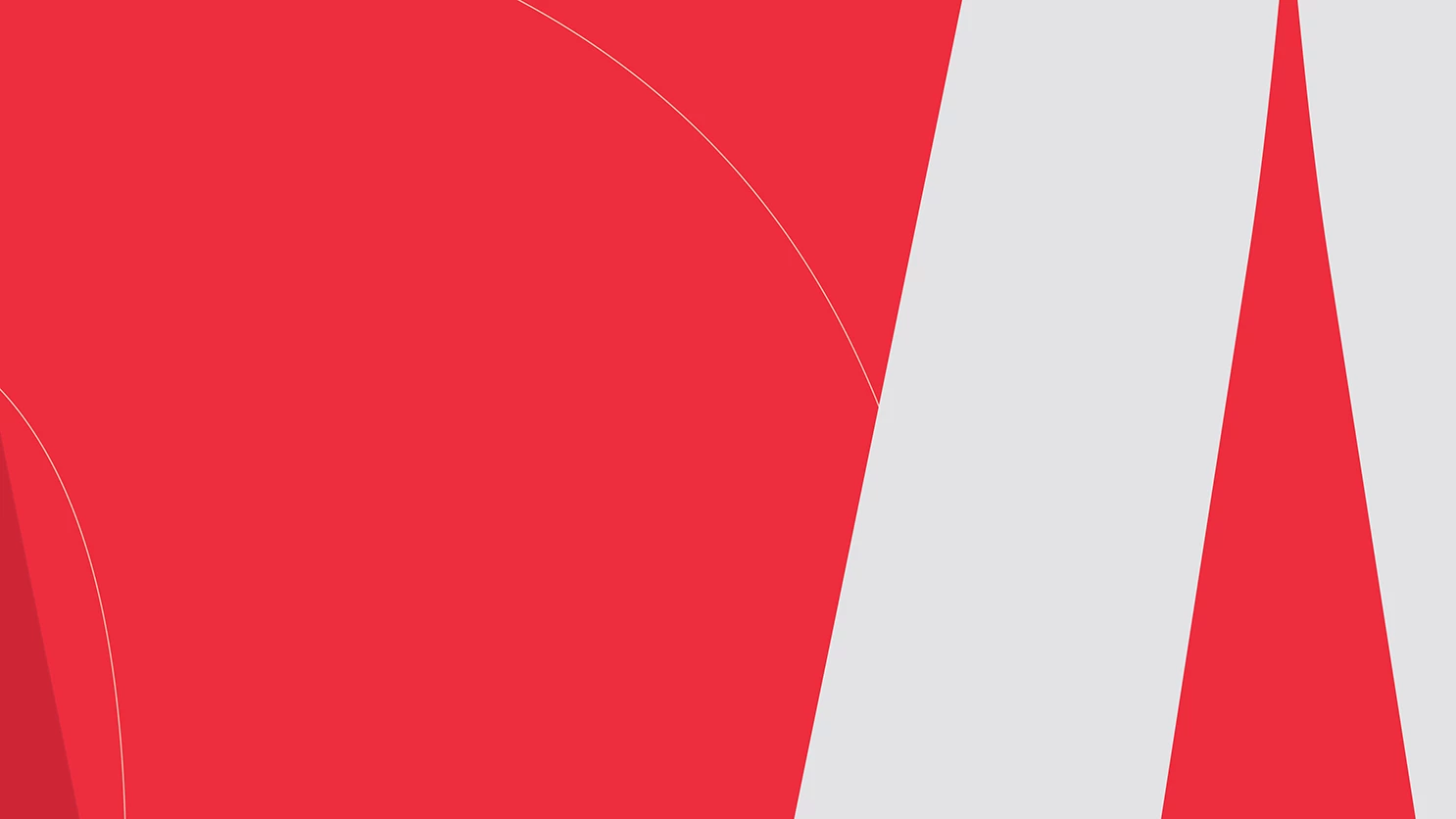
Article
NLP, LLMs, DeepML, and FastML: The AI Under the Hood of ABBYY Intelligent Document Processing
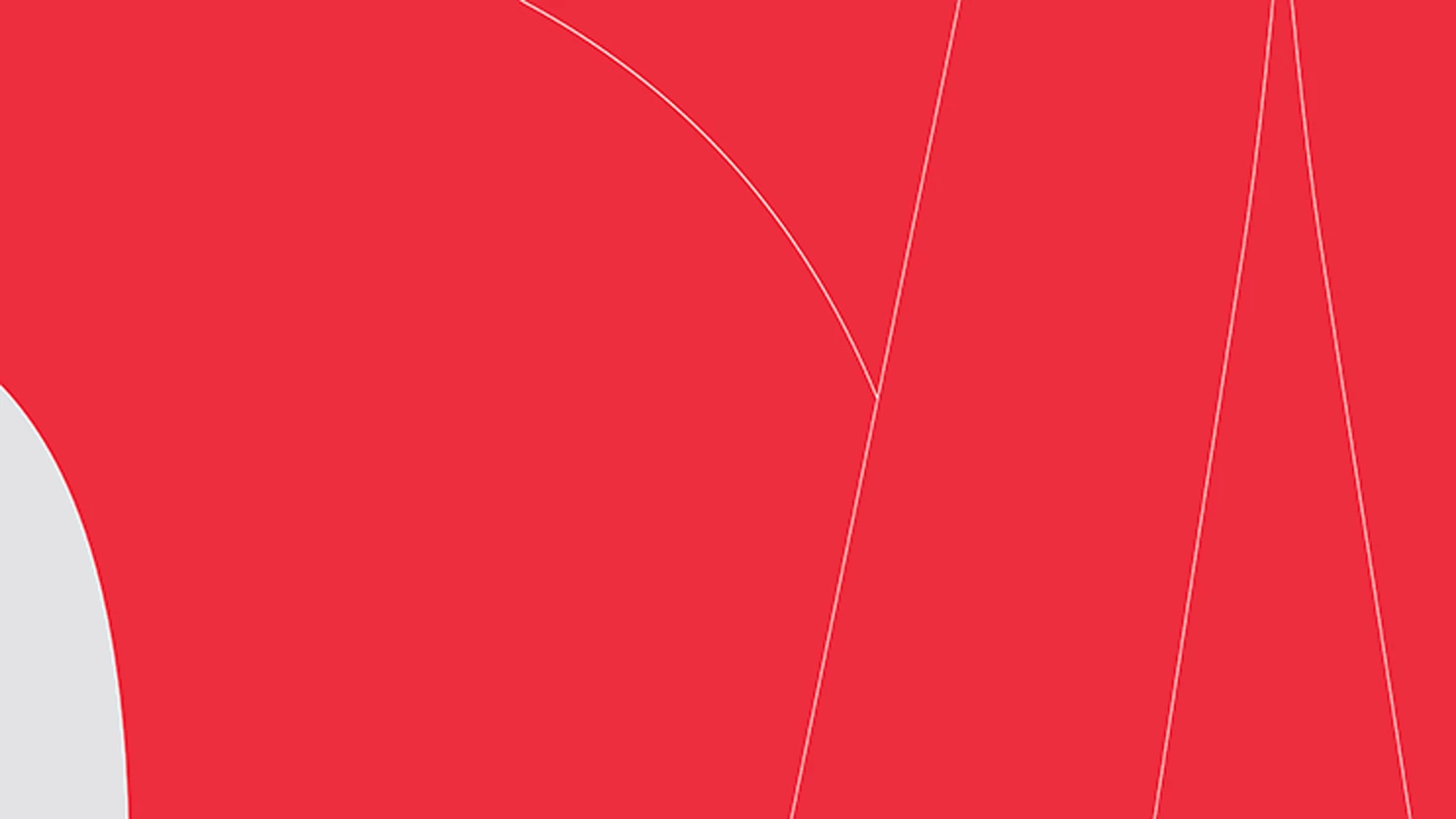
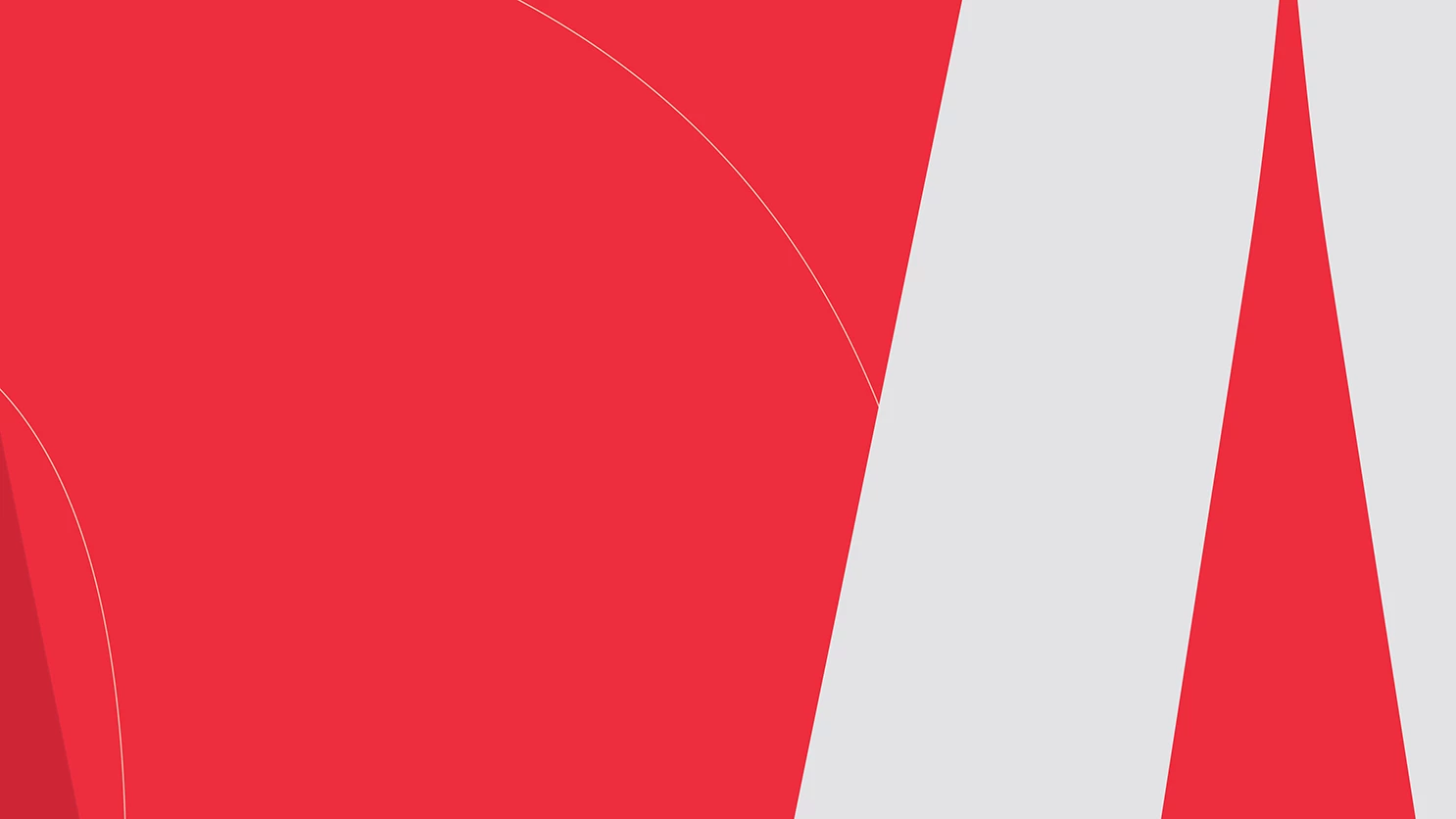
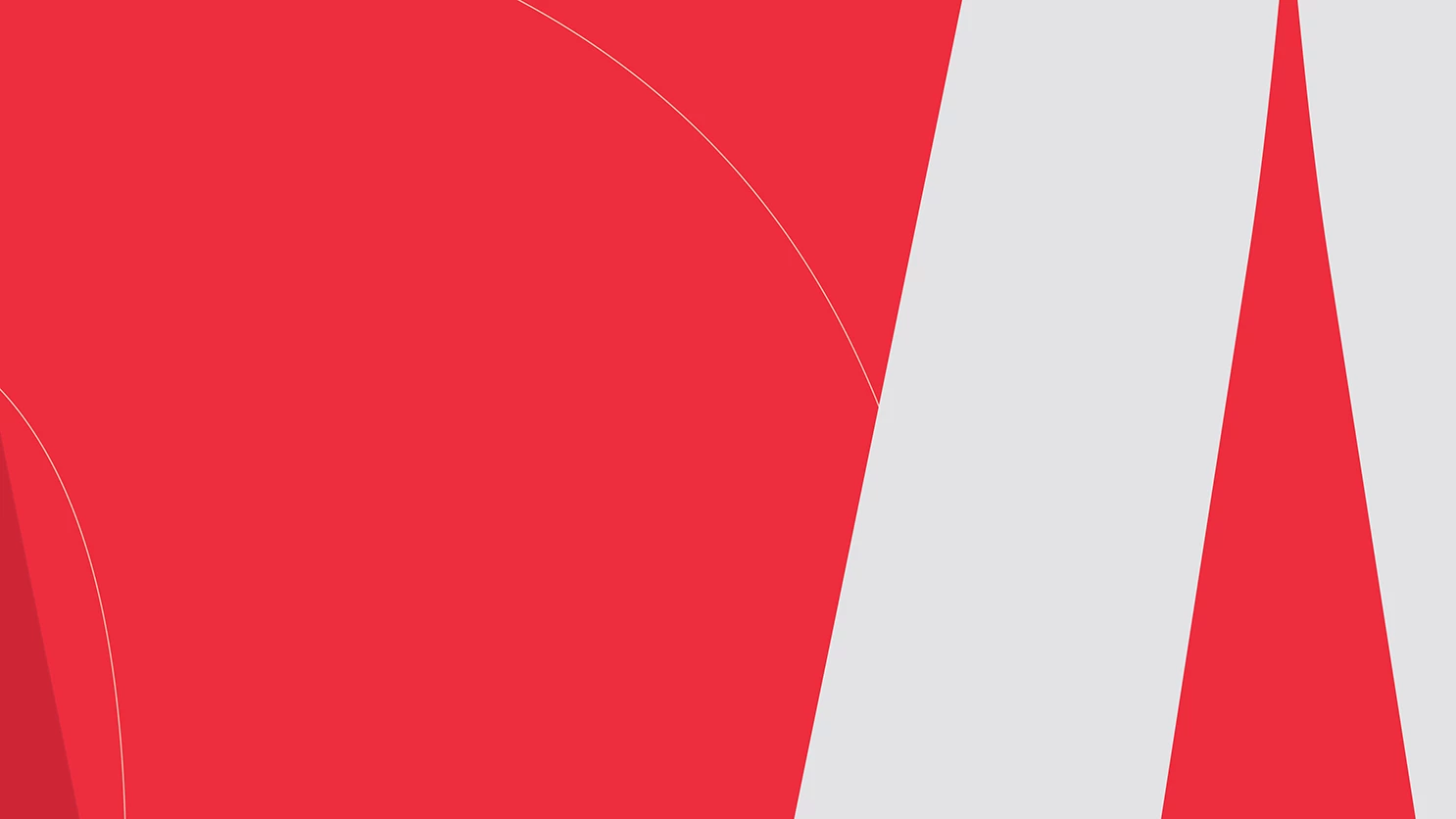
Article
NLP, LLMs, DeepML, and FastML: The AI Under the Hood of ABBYY Intelligent Document Processing
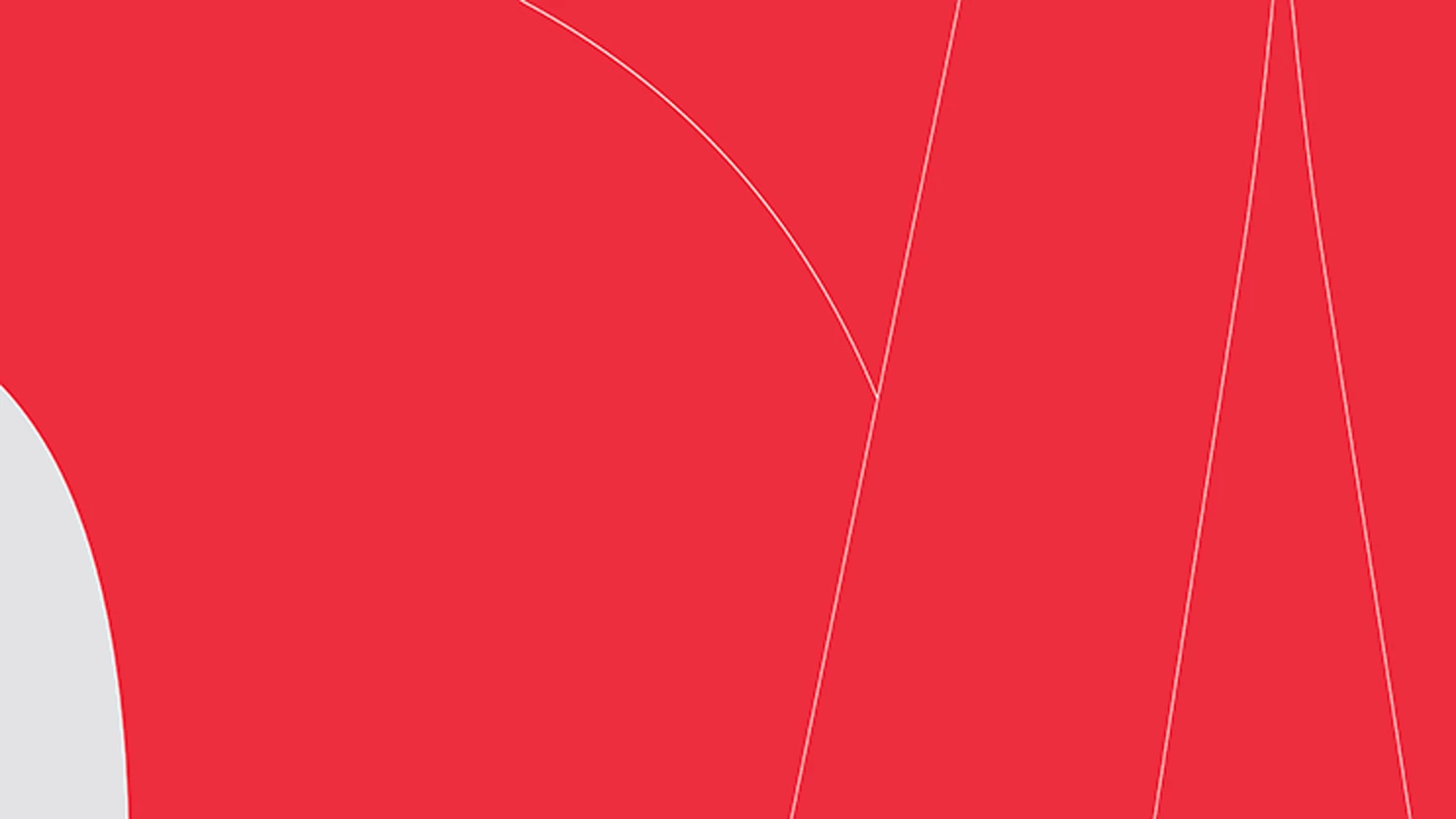
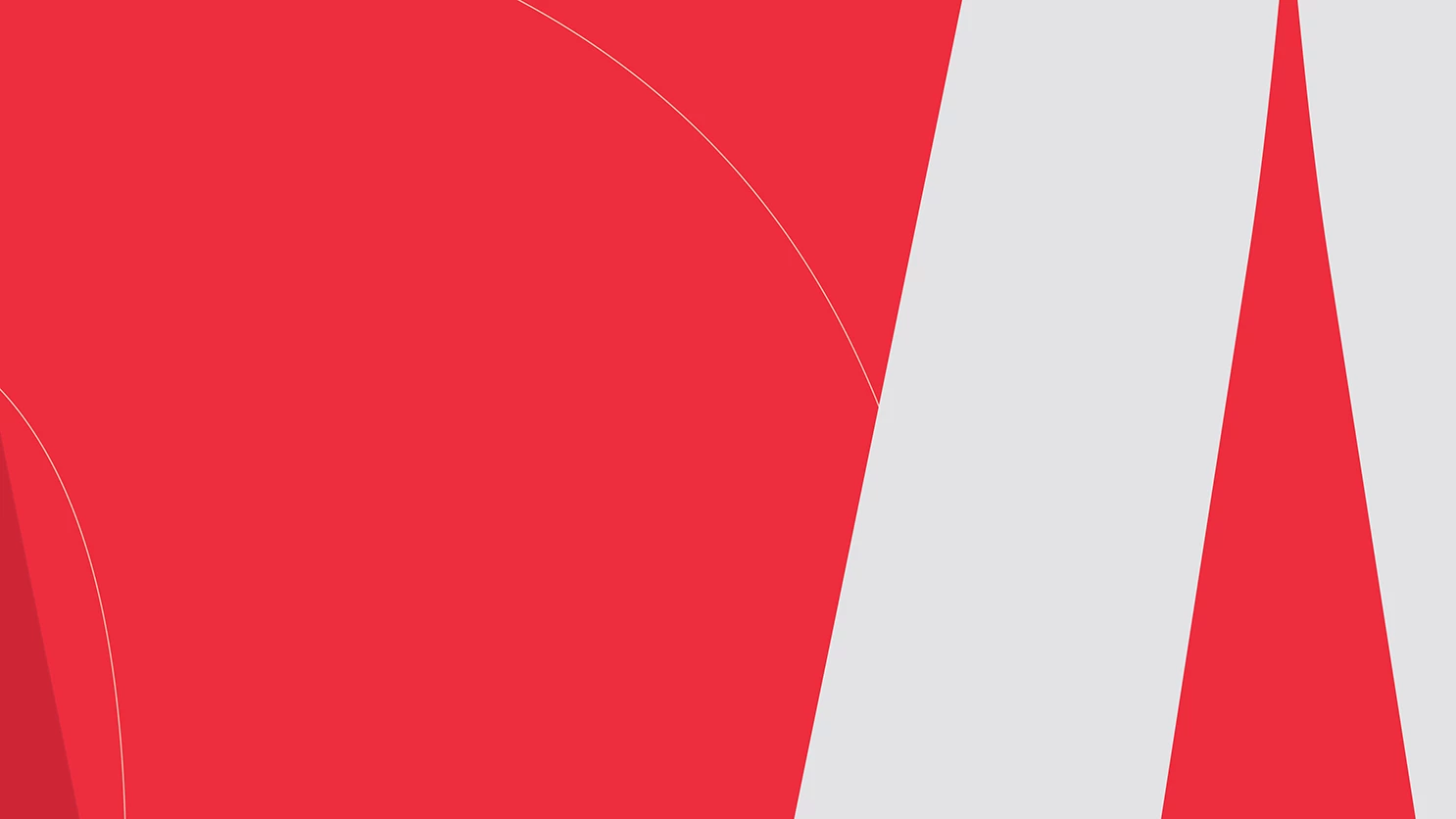
The perfect blend of Al
The effectiveness of RAG and similar generative Al initiatives rely on the underlying data quality. To realize the full potential of generative AI technologies, and deliver high-impact and ethically responsible outcomes, companies need to prioritize ongoing investment in acquiring, cleaning, and structuring data from their documents. This is made possible through ABBYY's Purpose-Built AI.
Make your data fluent in LLM
At ABBYY, we believe that data held in physical documents holds real value and useful insights when it’s used the right way.
We go beyond providing conventional document conversion services. We elevate your data, making it accessible and proficient in the intricate languages of LLMs.
Elevating conversion to transformation
Expertise in data extraction
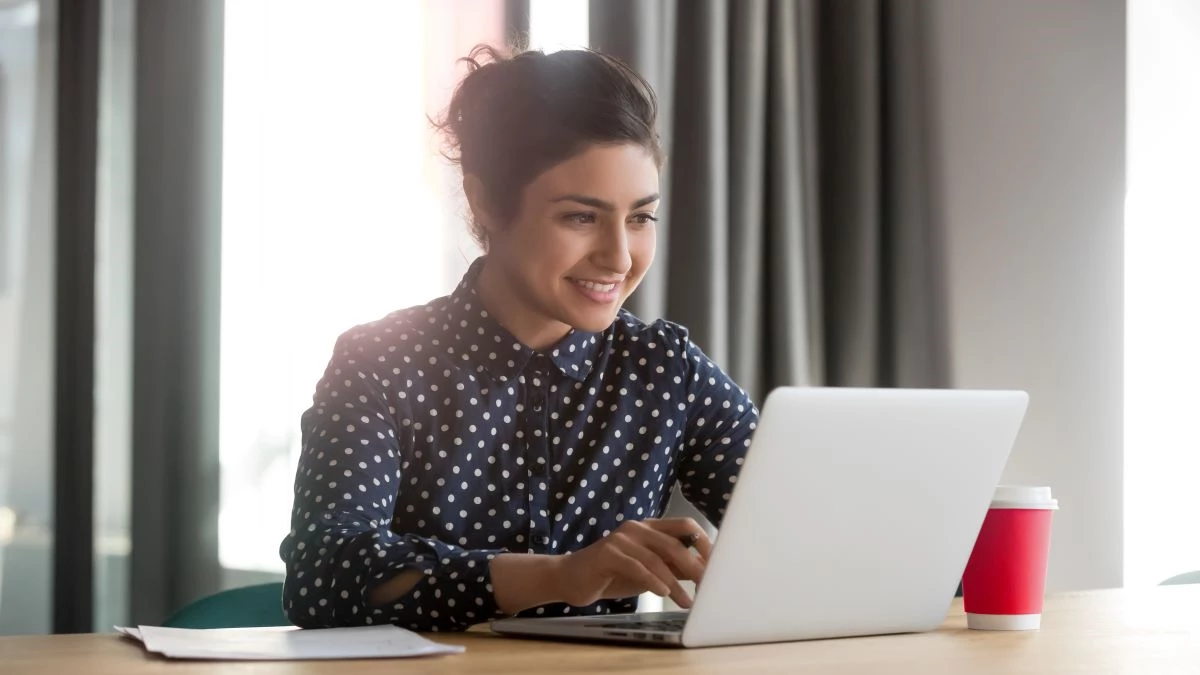
Why ABBYY?
Streamlined integration
Bespoke data solutions
Innovation partner
Discover how RAG can benefit your enterprise
Financial services
Purpose-built AI processes current, real-time market data. Improving the accessibility and relevance of this information can aid financial analysts in making prompt, well-informed decisions. Purpose-built AI can also support fraud detection by analyzing transaction data and highlighting potential fraud risks.
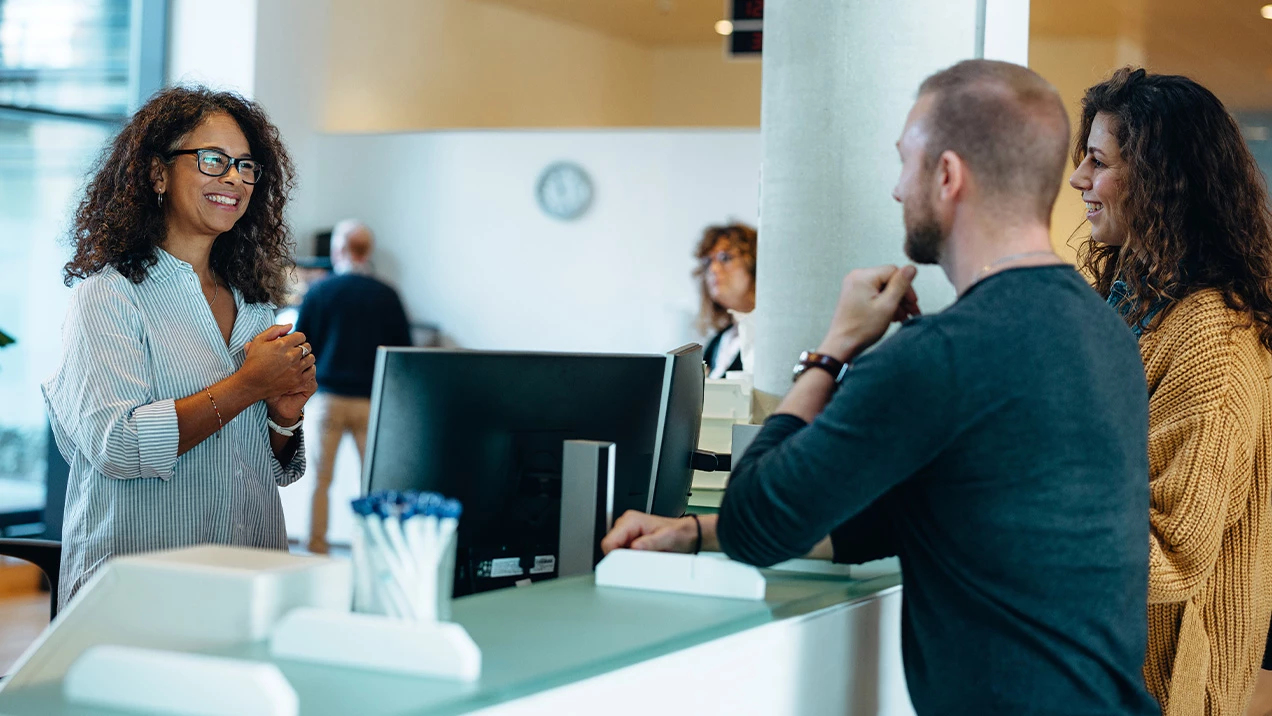
Healthcare
Purpose-built AI puts a vast bank of healthcare information at medical professionals' fingertips. Access to credible health research and case histories can support diagnoses and treatment of complex medical cases.
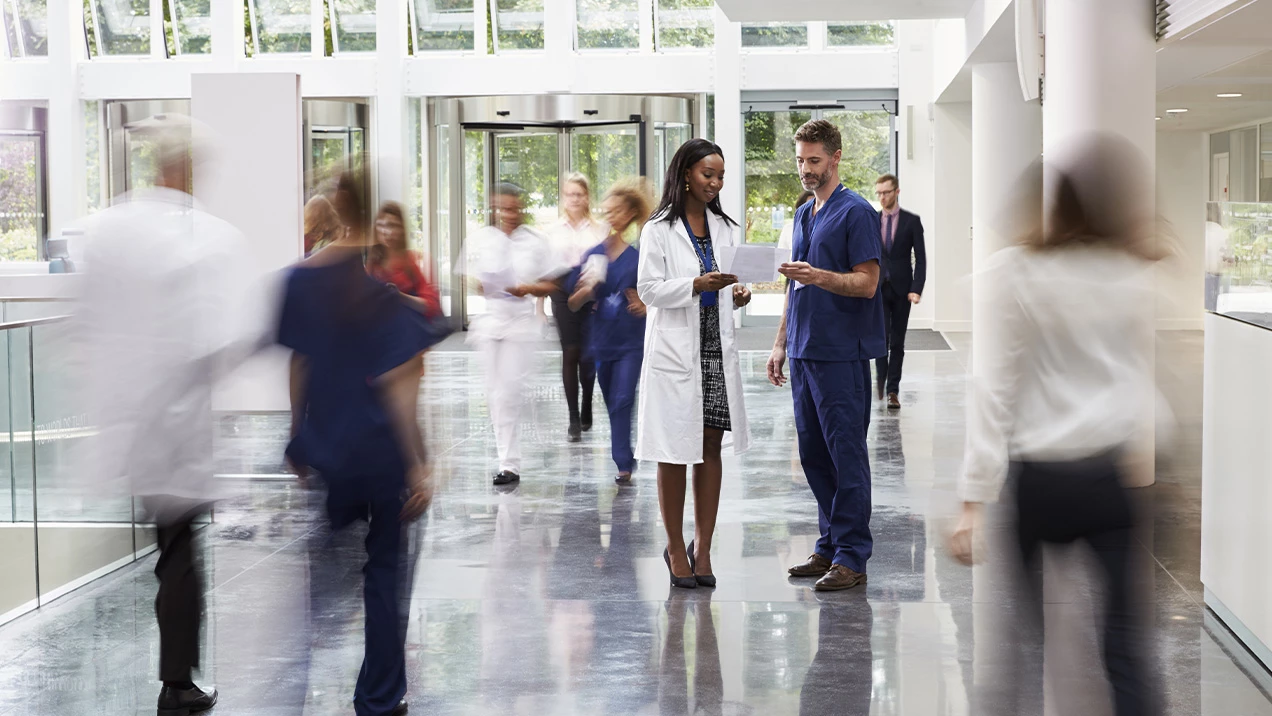
Education
Drawing from global teaching material can help education professionals create tailored content for their students. A personalized, student-centered approach can significantly enhance learning experiences and results.
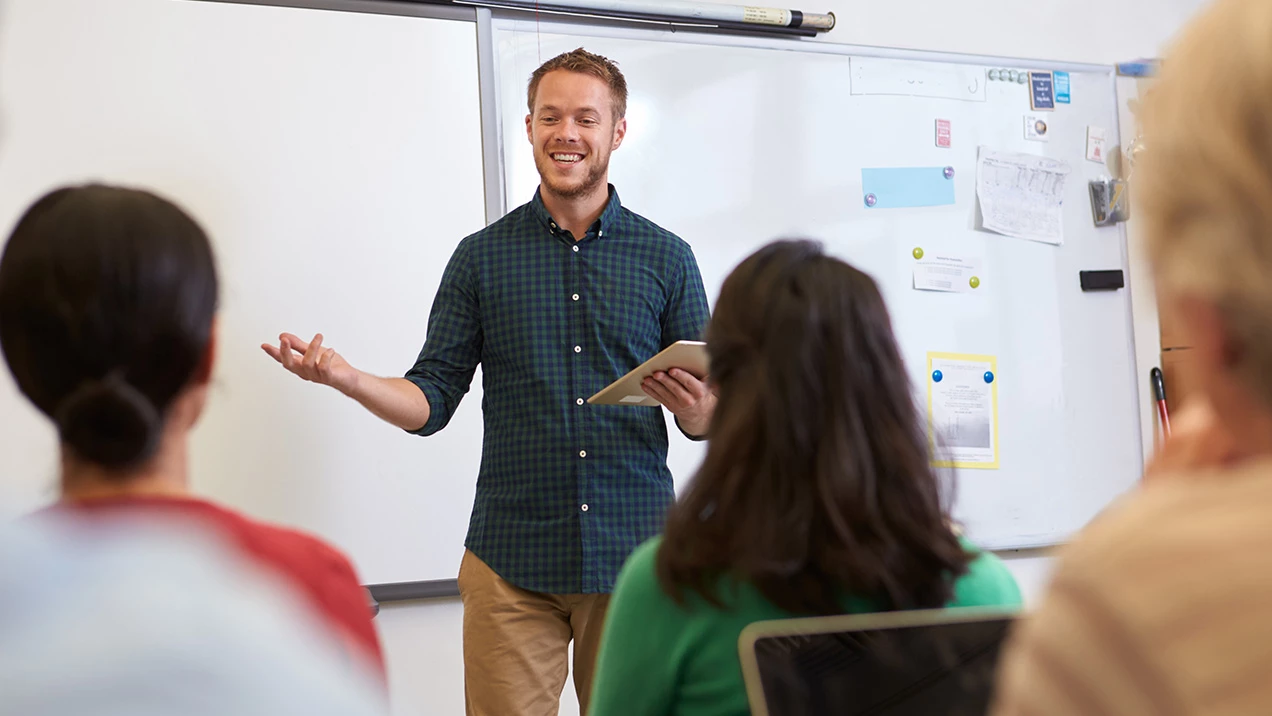
Financial services
Purpose-built AI processes current, real-time market data. Improving the accessibility and relevance of this information can aid financial analysts in making prompt, well-informed decisions. Purpose-built AI can also support fraud detection by analyzing transaction data and highlighting potential fraud risks.
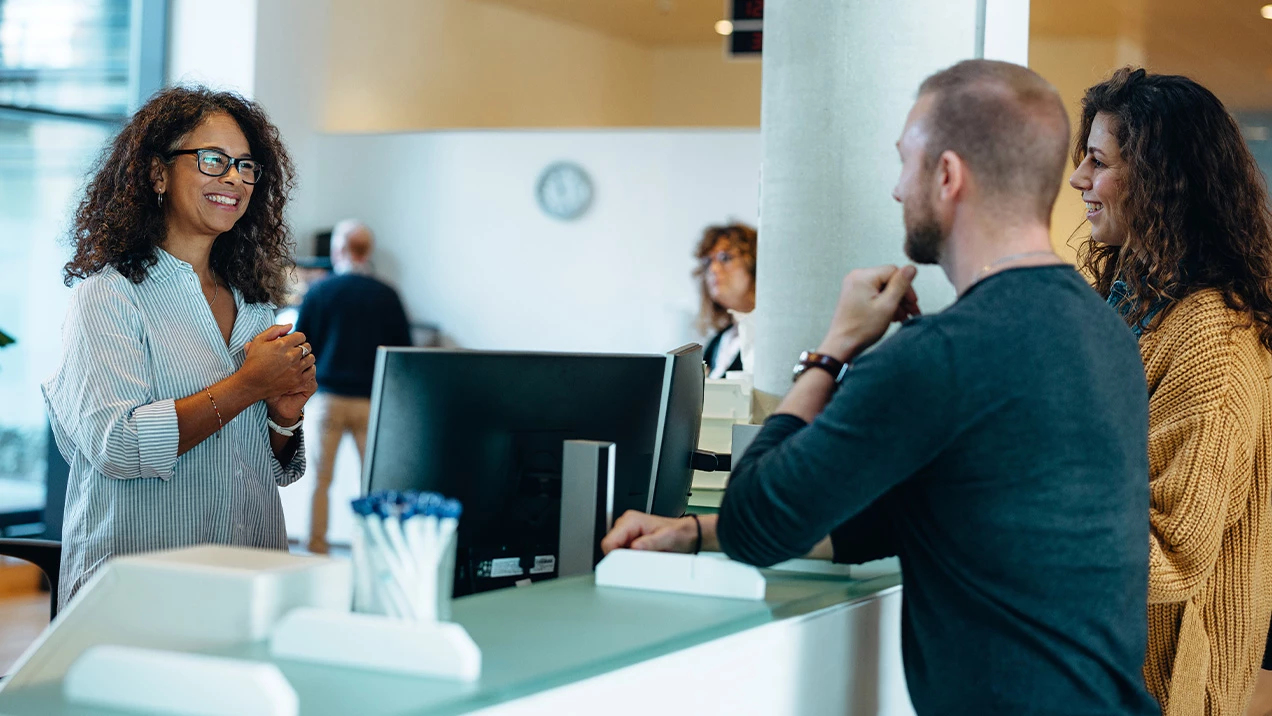
Healthcare
Purpose-built AI puts a vast bank of healthcare information at medical professionals' fingertips. Access to credible health research and case histories can support diagnoses and treatment of complex medical cases.
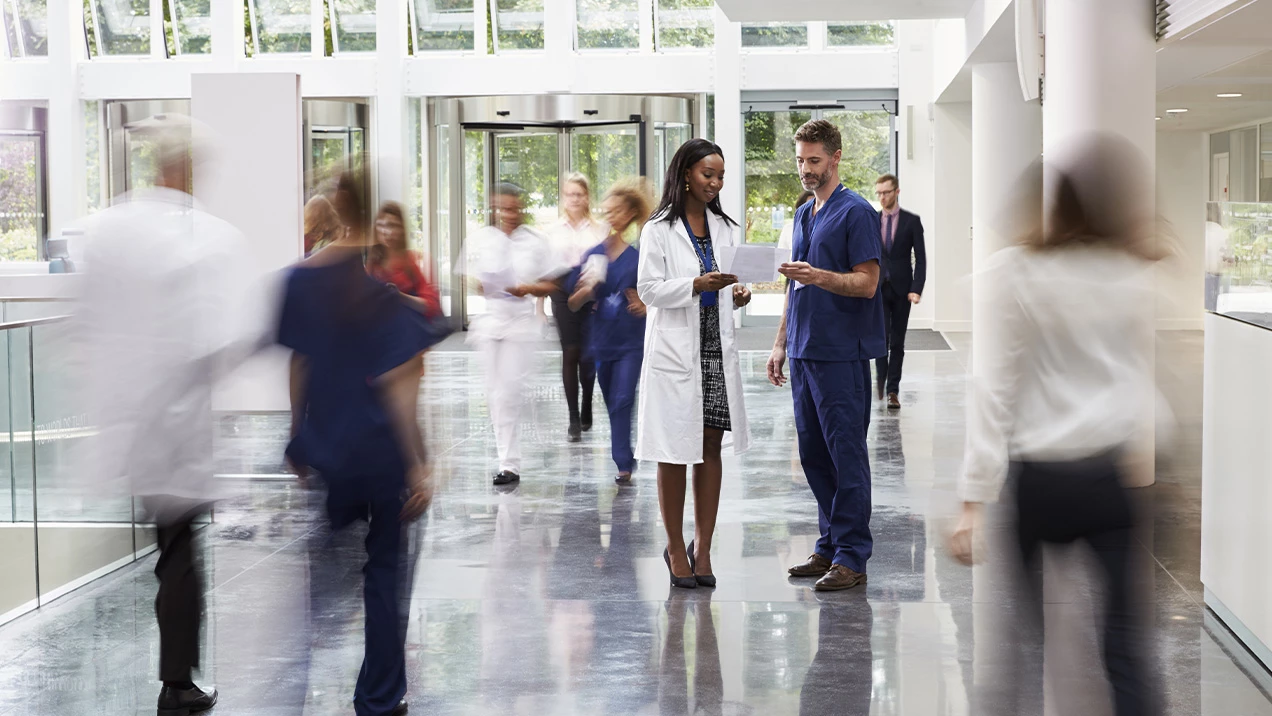
Education
Drawing from global teaching material can help education professionals create tailored content for their students. A personalized, student-centered approach can significantly enhance learning experiences and results.
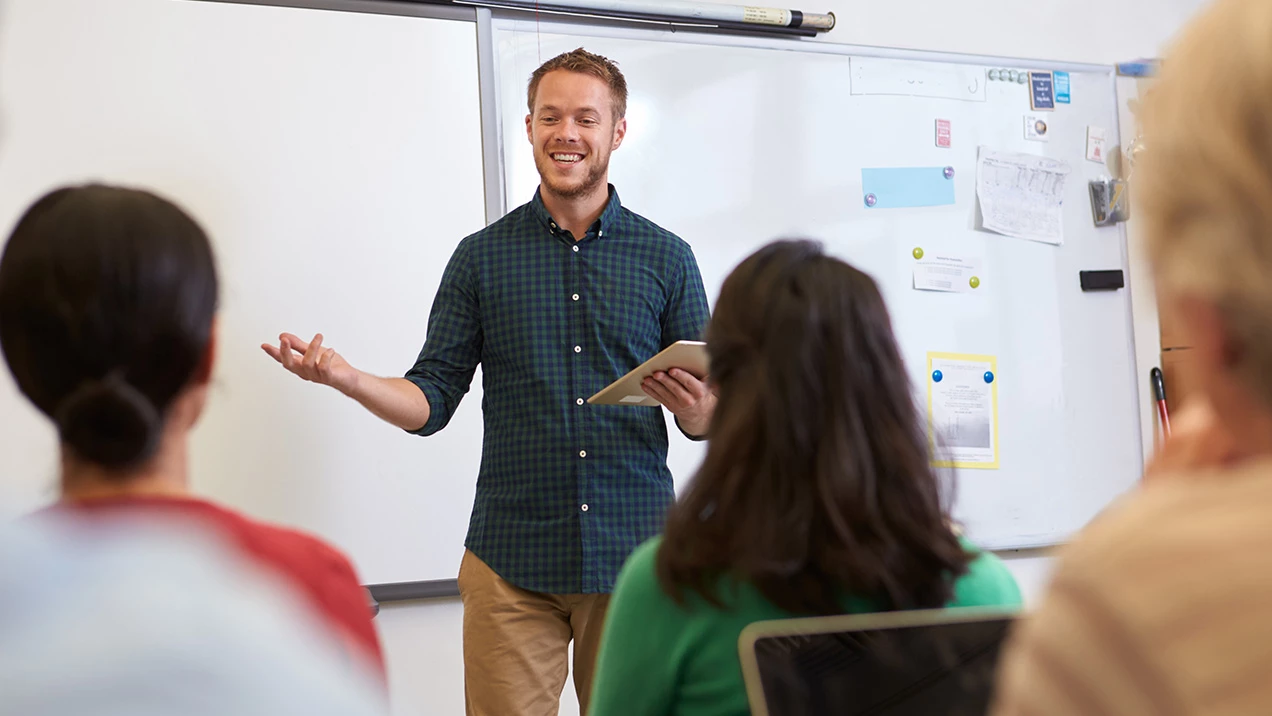
How does retrieval-augmented generation work?
Users typically give a large language model (LLM) a prompt or input, and receive a response based on its training data. RAG utilizes the user input to pull information from relevant external data sources. The user input and new information are then fed into an LLM to improve response quality. This process takes place in four steps:
- Compile external data
- Retrieve relevant information
- Improve the LLM input
Compile external data
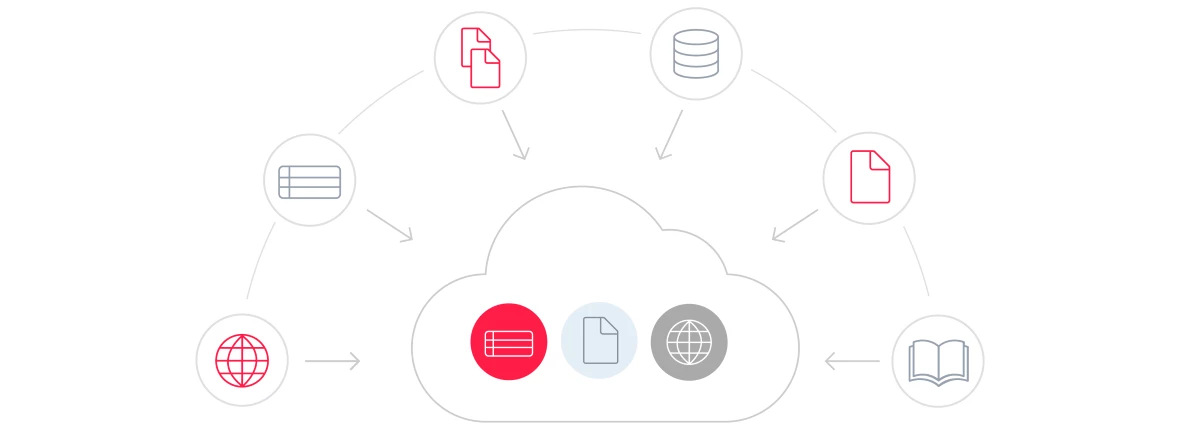
Retrieve relevant information
Improve the LLM input
We secure your business everywhere, so it can thrive anywhere
We've developed an integrated portfolio of purpose-built AI solutions to protect your business. Our security strategy, rooted in Zero Trust principles, empowers you to overcome uncertainty and global cyberthreats.
Learn more about ABBYY
ABBYY University
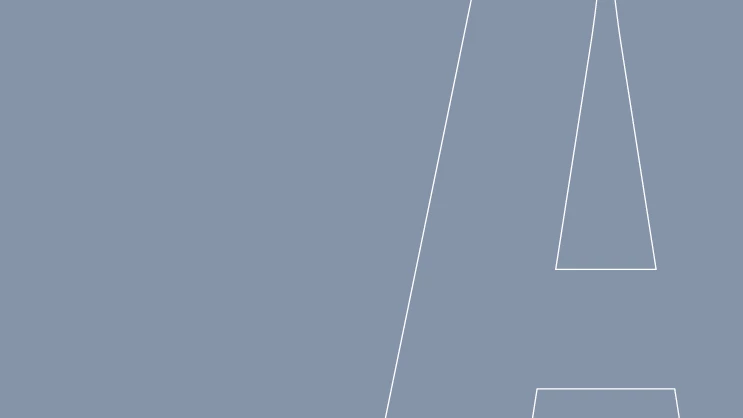
Vantage tutorial
The latest release of ABBYY’s intelligent document processing platform, Vantage, introduces a new ID reading skill. It supports classification and extraction of data from over 10,000 different document types in more than 190 countries.
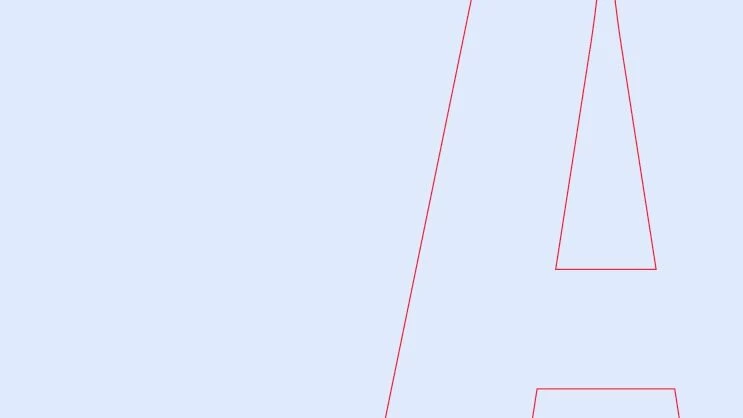
AI-Pulse Podcast
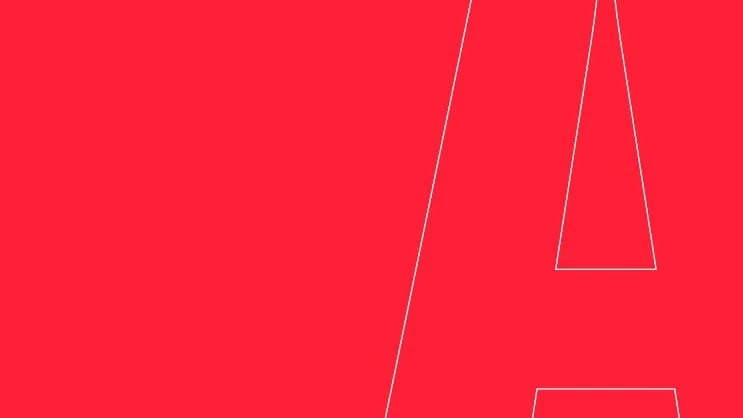
ABBYY University
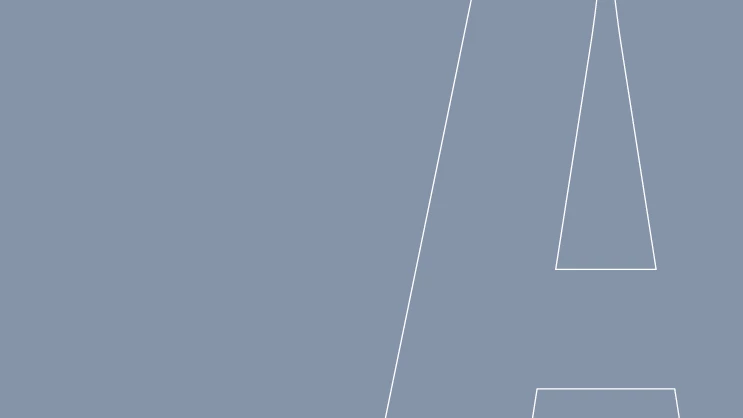
Unlock your AI potential with ABBYY
With more than 35 years of experience, we’re experts in intelligent document processing. We've perfected the development, implementation, and innovation of advanced algorithms and machine learning models. Our singular focus is to help you turn your inaccessible data into invaluable insights.
What are the benefits of partnering with ABBYY?
What are ABBYY's capabilities in document digitization?
How does ABBYY use natural language processing (NLP)?
Our NLP technology enables us to extract meaningful and contextual information from text-based content. NLP is a crucial tool for organizing unstructured data into actionable insights. With its capabilities, you'll be able to:
- Conduct advanced text analysis
- Evaluate sentiments
- Identify key entities
ABBYY Vantage combines NLP with RAG and other technologies such as OCR to offer comprehensive and relevant insights, beyond just document data.
Does ABBYY provide customized Al solutions?
Why is retrieval-augmented generation important?
RAG ensures LLMs retrieve information from accurate and relevant knowledge sources. LLMs are intelligent AI tools, but a crucial drawback is they may provide outdated information by drawing from static training data.
As a result, responses from conventional language generation models might be too generic or even inaccurate. RAG gives enterprises more confidence and control over generated outputs and the response generation process.
What are the benefits of retrieval-augmented generation?
There are three key benefits of RAG:
1. Relevant information
RAG provides current and reliable sources to LLMs, ensuring users receive the latest information.
2. Improved user confidence
RAG allows for source attribution, citations, and references. This increases users' confidence in generated responses.
3. Cost-effective training
RAG is a more affordable alternative to retraining a foundation model, making generative AI technology accessible.
What’s the difference between retrieval-augmented generation and semantic search?
Retrieval-augmented generation (RAG) and semantic search offer different approaches to information retrieval and generation. RAG combines language generation models with information retrieval techniques. It finds and integrates external data into large language models (LLMs) to improve response quality. In contrast, semantic search scans extensive databases to retrieve precise information. It accurately maps queries to relevant documents and returns specific text.
In summary, RAG prioritizes response generation from retrieved data, while semantic search focuses on delivering semantically relevant passages.